Lessons from Graduate School for the COVID Pandemic
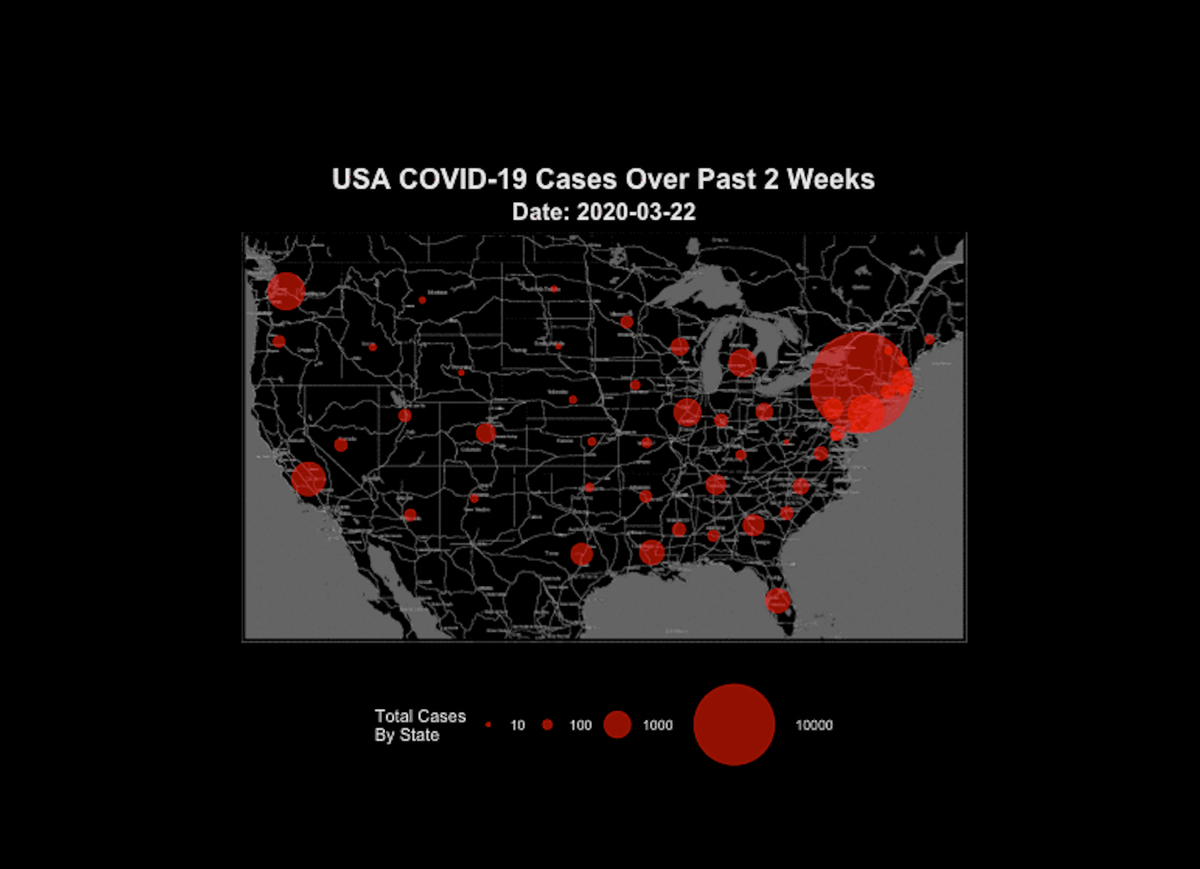
Data, medicine, and research have all of a sudden become part of every day life, and I have noticed the world is getting tripped up on a few lessons that every PhD student learns in their first few years of graduate school. Here is a cheat sheet to help you interpret the COVID-19 pandemic, and all the uncertainty around the data and studies we are seeing.
1. There is no such thing as perfect data.
There is only better data and worse data. I have seen people want to throw out all data on COVID-19 comparisons because none of the data is perfectly accurate. It is true that there is no database that has perfect counts of COVID-19 cases or deaths, and every plot of data you see likely has some (or many) inaccuracies in it. However, this does not mean it is useless. Some simple guidelines to follow are (1) find the most reputable data source you can, and (2) when you look at the data, take potential flaws into consideration, then (3) make the best conclusion you can with the data you have available, considering the flaws (Try “hmmm I think I see a trend here, but there is some uncertainty due to differences in testing” rather than “I know for sure that state A is doing a better job at social distancing than state B” or “this is all utter rubbish.”) This is the process used to interpret all data in science, not just rapidly emerging pandemic data. Yes… some data is so flawed that it should be thrown out because it’s trash, but if your standard for throwing out data is if it contains any flaws or inaccuracies at all, you will be throwing out pretty much every data set in existence.
2. It is easy to only pay attention to the data that supports your hypothesis (and ignore
the data that goes against your hypothesis).
It is easy, but oh so wrong. This is why I personally remained skeptical of the anecdotal evidence for hydroxychloroquine/azithromycin efficacy against COVID-19… we have heard reports from physicians saying that it does work, which is exciting. But is it possible that only the positive stories are getting circulated? If a doctor tries this drug combination and it doesn’t work, are they going to be interviewed by the evening news or shared all over facebook? Probably not. Likewise, early on there were multiple small studies on whether hydroxychloroquine/azithromycin works in humans… with conflicting results. Should we only pay attention to the studies that say it works, and ignore studies that say it doesn’t work? Nope. We have to judge each of these studies based on the quality of the study, not on whether it gives us the answer we want.
3. It is easy to get fooled by early data.
This one I think nearly every scientist has been guilty of at one time or another. You start an experiment, have a few samples, and it looks like there is an amazing result! You get so excited and want to publish immediately! You’re about to win the Nobel Prize!!! However, as you do the more robust experiments (with more samples), those exciting results start to look less exciting. This has happened to me on multiple occasions in my research (minus the Nobel Prize bit). The need for an adequate sample size (with proper controls) is a real thing… but I think it’s hard to appreciate how important this is until you’ve been fooled by your own data.
4. There is a big difference between studies done in human cells in a dish (in vitro), in
animals, and in real breathing humans.
The vast majority of treatments that look promising in a dish or in animals end up failing in humans. If you’re curious why this is (besides the obvious fact that neither cells in a dish nor mice can capture the full biological complexity of a human being), check out the book Rigor Mortis: How Sloppy Science Creates Worthless Cures, Crushes Hope, and Wastes Billions (it’s not nearly as depressing as that title sounds). But the tl;dr version is — wait for the human clinical trials to decide if a drug really works.
5. Scientific and medical expertise does not readily transfer fields.
An expert in physics is not the right person to critique an epidemiological model predicting how many COVID-19 cases there are going to be. Not all medical doctors are trained to do scientific research and design experiments. And a researcher (PhD) may know everything there is to know about the biology of a specific virus, but they do not know how to treat a decompensating ICU patient sick with that virus. Do your best to listen to people who are speaking out of their expertise and who know their own limitations.
6. It takes a long, long time to be sure of anything in science.
Most of the things we “know” in science are based on hundreds (if not thousands) of studies from research that has been building for decades. We scientists do get excited about a single new study that shows something surprising and new, but we always take it with a big grain of salt, because we know that no single study is perfect. Instead we rely on evidence from many studies before a scientific hypothesis is converted to a scientific fact.