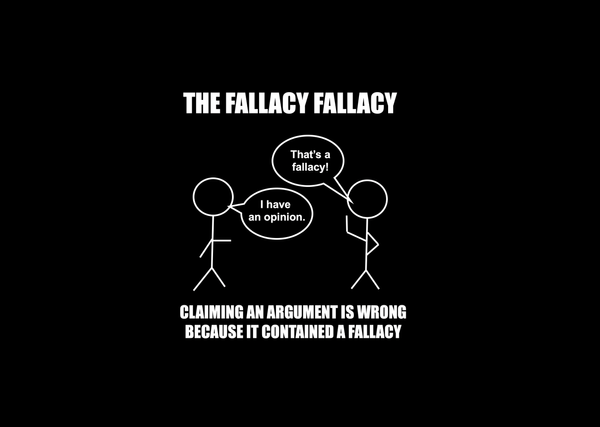
COVID
Logical Fallacies in the Time of COVID
As I’ve gone into the world of facebook comments, I have noticed that many people trying to make sense of the pandemic (myself included) are running into a number of logical fallacies. As you listen to other people’s views and form your own, try to keep these fallacies