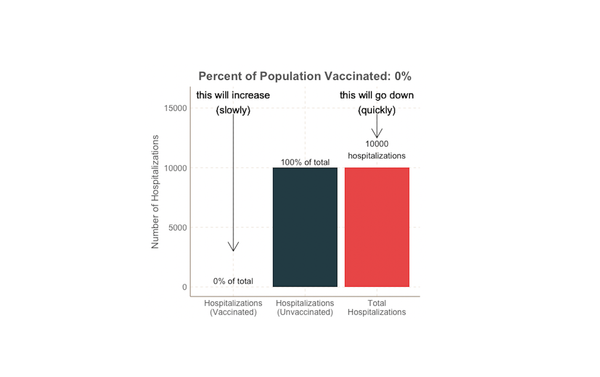
COVID
Some vaccinated people are getting COVID. What does this mean?
Delta is here and headlines are reporting the rise in new cases and hospitalizations, including some who have been fully vaccinated. What does this mean? Does the fact that some vaccinated people are getting sick mean the vaccines aren’t working? Some breakthrough infections are expected Thankfully, no. Even if